Explainable Attribute-wise Fashion Compatibility Modeling
Abstarct
Fashion compatibility modeling (FCM) which aims to evaluate the compatibility of fashion items, e.g., the t-shirt and trousers, plays an important role in a wide bunch of commercial applications including clothing recommendation and dressing assistant. Recent advances in multimedia processing have shown remarkable effectiveness in accurate compatibility evaluation. However, the existing methods typically lack of explanations for the evaluation, which are of importance for gaining users' trust and improving the user experience. Inspired by the fact that fashion experts explain a compatibility evaluation through matching patterns across fashion attributes (e.g., a silk tank top cannot go with a knit dress), we propose to explore the explainable FCM by leveraging comprehensible attribute semantics. As the main contribution, we devise an explainable solution, named ExFCM for FCM, which simultaneously generates the compatibility evaluation for input fashion items and explanations for the evaluation result. In particular, we first design a new neural network operator to learn the attribute-wise representation for each input fashion item. Additionally, we develop an explainable scheme to infer attribute-level matching signals between fashion items. Furthermore, the matching signals are dynamically aggregated into an overall evaluation. Note that ExFCM is trained without any attribute-level compatibility annotations. Extensive experiments on a real-world dataset verify that ExFCM generates evaluations more accurately than several state-of-the-art methods, together with reasonable explanations. Codes will be released once acceptance.
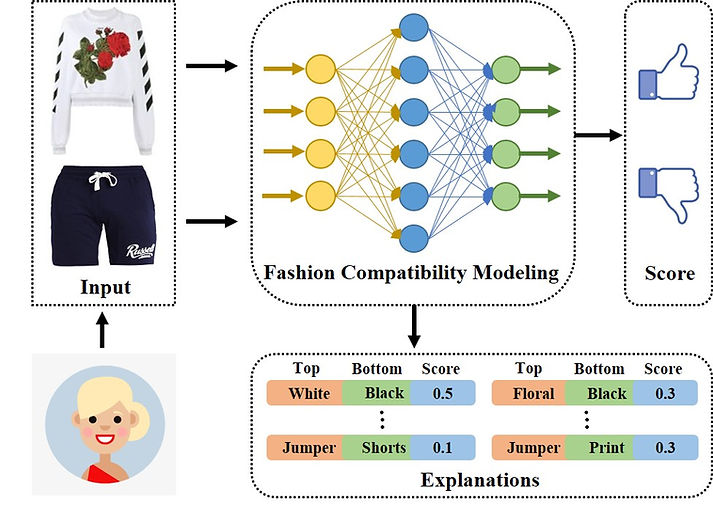
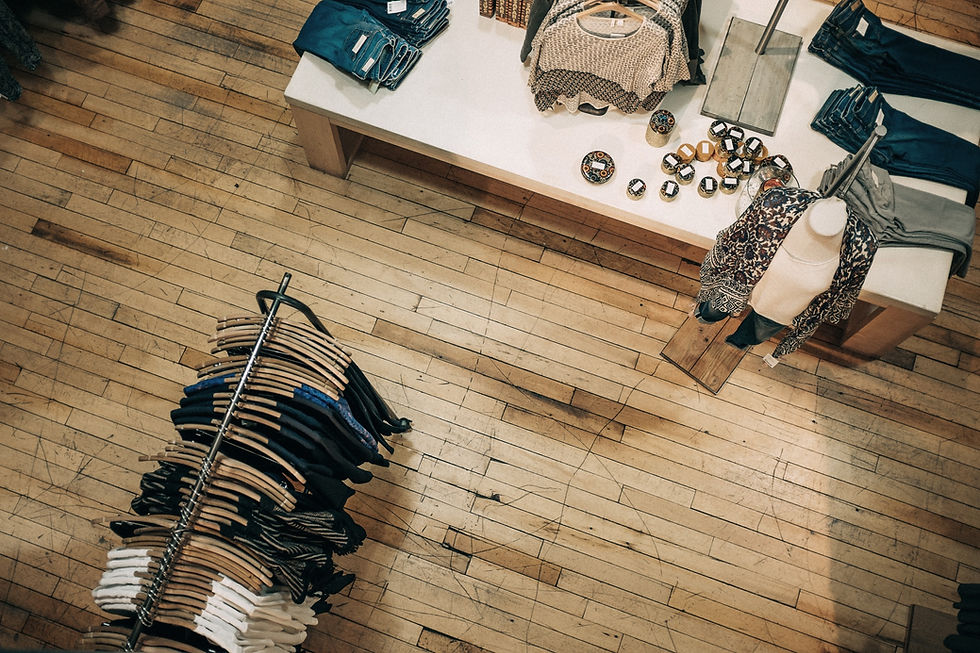
Contributions
We propose an explainable method (ExFCM) for fashion compatibility modeling, which generates attribute-wise explanations for compatibility evaluation.
The proposed model is capable of inferring attribute-level matching signals between fashion items without any attribute-level compatibility annotations.
Extensive experiments on a real-world dataset validate that ExFCM generates evaluations more accurately than several state-of-the-art methods, together with reasonable explanations.